5 Lesser Known Facts about The Ideal Backtesting Setup
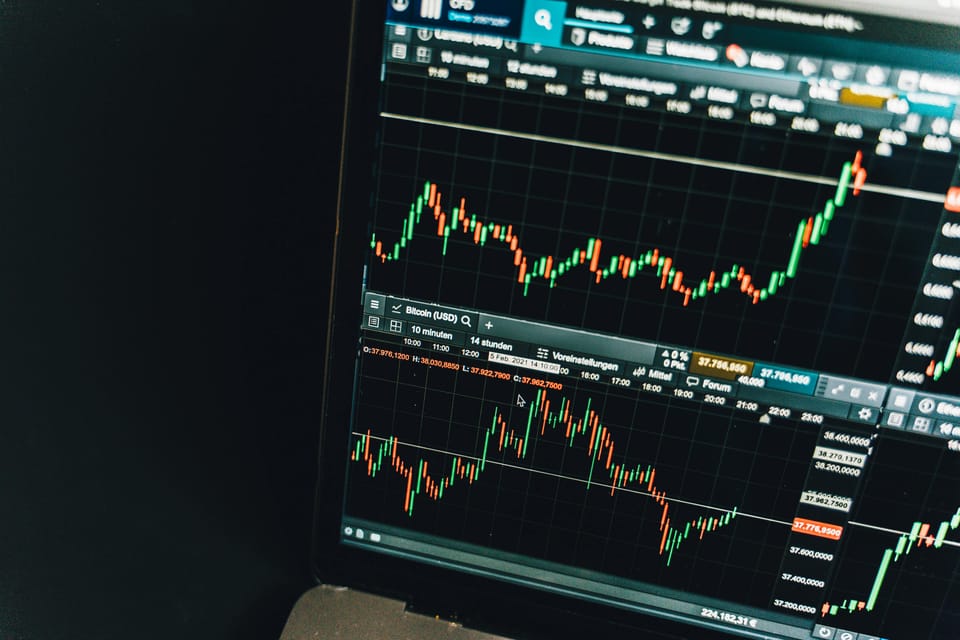
A well-designed backtest is the cornerstone of any sound trading strategy.
But what if there's more to Backtesting than meets the eye?
In this blog, we will discuss the common backtesting practices to explore five lesser-known facts about the ideal backtesting blueprint.
We'll see how seemingly minor details can significantly impact the reliability of your results and, ultimately, the success of your trading journey.
To ensure an exceptional backtesting approach, always consider the following key points:
1) It Captures All Market Cycles
Ok,
So this is the first point to consider.
Ideal backtested data should span a significant period that encompasses a variety of market conditions (bull runs, bear markets, corrections).
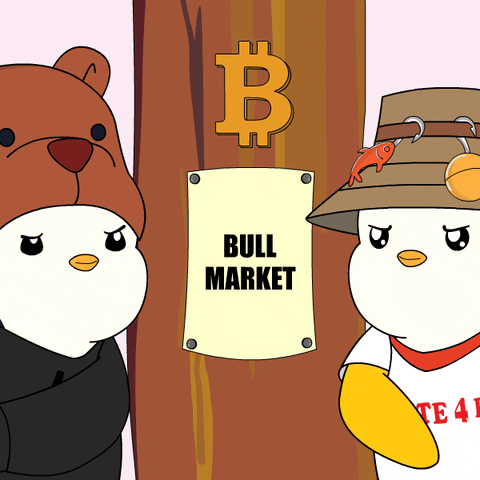
This allows you to assess your strategy's effectiveness across different scenarios, reducing the chance of mistaking a fluke for a sound approach.
Why?
Because markets experience distinct phases that challenge strategies in different ways:
For example, Soaring Bull Markets, characterized by rising prices and optimism, can make many strategies look successful. Your backtest should assess how your strategy handles this exuberance. Can it capture profits while avoiding overexposure as the market climbs?
Similarly,
Bear markets with plummeting prices and investor fear are inevitable when you trade live. Here, your backtest reveals your strategy's resilience.
Does it manage risk effectively and identify potential short-selling opportunities during downturns?
Not only in Bulls and Bears, but your trading strategy should also show how your strategy will perform during Corrections.
See, Markets sometimes go up in a straight line. Corrections and temporary price drops can present entry points for skilled traders. It would help if you showed how your strategy handles these corrections.
It should answer the question,
Does it capitalize on these short-term buying opportunities or succumb to panic selling?
Let's take an example.
Let's say you've developed a trend-following strategy based on moving averages. It performs well in a simulated bull market (rising prices). However, you need to figure out how it would handle other market conditions.
So, what you do is to test the strategy in different market conditions
You can do the following to test your strategy in all market cycles.
Bull Market (2003-2007): The strategy can be applied to historical data from the mid-2000s bull market, which confirms its ability to capture profits during an extended uptrend.
Bear Market (2008): You can also test the strategy during the 2008 financial crisis. This reveals how the strategy handles sharp downturns and the potential for losses. Analyze how quickly the strategy generates exit signals to limit losses.
Consolidation Period (2010-2011): Similarly, you can apply the strategy to a period of sideways movement (consolidation) after the crash. This assesses the strategy's ability to identify and avoid unnecessary trades during flat markets that lack clear trends.
By testing across these diverse market conditions, you gain a more comprehensive understanding of the strategy's strengths and weaknesses.
You may come to know that.
The strategy might excel at capturing trends in bull markets, as initially observed in simulations.
Or you may observe its Weaknesses. For example, The strategy might struggle during bear markets, potentially generating significant losses. It might also generate excessive trades during consolidation periods, leading to unnecessary transaction costs.
This gives you the chance of Refinement!
Based on your analysis, you can refine the strategy, for example
By Introducing filters. Adding filters to the moving average strategy may avoid generating excessive trades during consolidation periods.
The Refinement may also help you make stop-loss adjustments. Tightening the stop-loss parameters during bear markets may limit potential losses.
In a nutshell,
By exposing your strategy to this spectrum of trading conditions, you gain invaluable insights. You can differentiate between a strategy that thrives only in specific market environments and one with broader adaptability. This reduces the chance of mistaking a lucky fluke for a truly robust trading approach.
2) It Includes Adjustments like Delisting Merger & Bankruptcy
A problem overlooked by even experienced traders!
When backtesting a trading strategy, incorporating real-world market events like company delistings, mergers, and bankruptcies is crucial for obtaining a realistic picture of its performance. Here are some specific examples of how ignoring these events can skew your results:
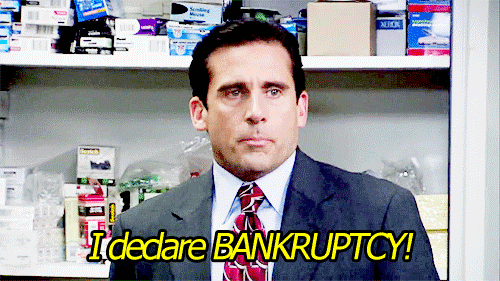
Scenario 1: Short Selling Strategy with Delisted Companies
Let's say you develop a short-selling strategy that identifies overvalued stocks and profits from their price declines. Backtesting with readily available data shows consistent profitability.
However, there is one little problem!
The data doesn't include companies that have been delisted (removed from the stock exchange).
Now, with this problem in your data, Imagine the strategy identifies Company X for shorting due to its seemingly inflated price. However, shortly after, Company X went bankrupt, and its stock price plummeted to zero. This would be different from your backtesting data.
So, what does it lead to?
Yes, leading to an overestimation of the strategy's potential returns
It's because Ignoring delistings paints an overly optimistic picture. Your strategy might appear highly profitable because it "benefits" from companies disappearing entirely, whereas, in reality, you wouldn't be able to profit from their stock price decline.
Scenario 2: Mean Reversion Strategy with Mergers
This is another kind of error you may encounter in your data.
Let's say you implement a mean reversion strategy that buys stocks that have fallen below their historical average price, expecting them to rebound. Your Backtesting will show positive results.
However, what if the data needs to account for mergers between companies?
With this error in your data, Imagine the strategy identifies Company Y for buying because its price has dropped significantly. However, In reality, company Y merges with another company, creating a new entity with a different stock price.
So, what will the impact of Backtesting be on this data?
Ignoring mergers can lead to misinterpretations. Your strategy might appear effective at identifying undervalued stocks, but the post-merger stock price of Company Y might not necessarily revert to the pre-merger price.
Scenario 3: Long-Term Investment Strategy with Bankruptcies
Now, here is another interesting scenario,
Imagine you develop a long-term investment strategy that focuses on buying and holding promising companies for their growth potential. Backtesting shows steady returns.
But again, there is a little problem in your data,
The data doesn't account for companies going bankrupt.
With this error in your data, Imagine the strategy identifies Company Z as a long-term investment based on its strong fundamentals. However, Company Z later declared bankruptcy, and its stock became worthless.
Did you notice the impact?
Ignoring bankruptcies can underestimate the strategy's risk. Your backtesting results might show consistent growth, but you need to reflect the potential for a company to go bankrupt and wipe out your investment.
So, How to Address these Issues?
Well, You can have a few options,
Number 1 is to use comprehensive Data Feeds. Look for data providers that offer historical data with adjustments for delistings, mergers, and bankruptcies.
If comprehensive data isn't readily available, consider simulating these events in your Backtesting. For example, assume a delisted company's price drops to zero at the delisting date.
For mergers, You can research the merged entity's new stock price and adjust your backtesting results accordingly.
The bottom line is,
By incorporating real-world market events, you gain a more accurate picture of your strategy's potential performance and can make informed decisions about its viability in the real market.
3) It's not just Too Simple Risk Management.
Sound Risk Management measures aim to protect your capital from excessive losses. However,
Most beginner traders need to consider an overall risk management strategy before using risk management tools that are too simple.
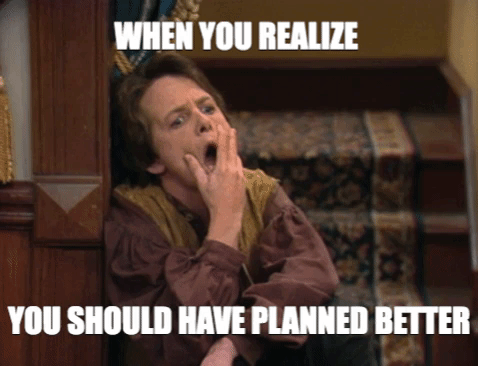
How? Let's see.
Let’s say You developed a swing trading strategy that identifies stock price swings with a potential 5-10% profit.
But,
Initially, you relied on a simple rule of "never losing more than 3% per trade."
You see, this kind of static rule for risk management has some Limitations!
Isn't it Overly Cautious?
If you're aiming for 5-10% gains, a 3% loss limit might prevent you from capturing profitable opportunities.
because,
A trade setup with a potential 10% profit and a 3% stop-loss distance (the distance from your entry price to the stop-loss level). Translates to a risk-reward ratio of 3:1 (potential loss / potential gain). A risk-reward ratio this low might not be attractive for many traders, as the potential reward needs to justify the potential risk sufficiently.
It also Ignores Position Sizing. The static rule doesn't consider how much capital you risk on each trade. You could still risk a significant portion of your capital on every trade, even with a high win rate. This can quickly deplete your capital if you experience a losing streak.
So, How do we solve this problem?
To address this problem, you need to have a refined risk management approach.
In this refined approach, You move beyond the static 3% loss limit and establish a dynamic risk tolerance range of 1-2% per trade. This allows you to adjust your risk based on the specific trade setup.
For trades with a higher potential profit (closer to 10%), you might be comfortable risking closer to 2% of your capital. This allows you to capture potentially larger gains while still managing risk.
Similarly, For trades with a lower potential profit (closer to 5%), you might choose to risk only 1% of your capital. This reflects the lower potential reward with a smaller potential loss.
You may also need to adjust Position Sizing based on Stop-Loss
You may determine your stop-loss placement will be 2% below the entry price for this strategy. This stop-loss distance helps define your position size.
The formula is:
Position Size (%) = Risk Tolerance (%) / Stop-Loss Distance (%)
Let's say you choose a 1% risk tolerance for a specific trade setup with a 2% stop-loss distance:
Position Size (%) = 1% / 2% Position Size (%) = 0.5%
This means you would only risk a maximum of 0.5% of your total capital on this particular trade.
Also,
you may need to cater for Stop-Loss Orders
To do risk management using stop loss, You may set stop-loss orders 2% below your entry price to automatically exit losing positions and limit potential losses to 2%. This removes the emotional burden of manually exiting losing trades and ensures your risk stays within your defined parameters.
And last,
you may opt for Diversification. You may allocate a portion of your capital to this swing trading strategy while also holding some other long-term assets in a different asset class, like bonds. This helps diversify your overall portfolio risk.
4) It Avoids Highly Correlated Assets.
Correlation measures the degree to which two assets tend to move in the same direction.
A correlation coefficient of +1 indicates a perfect positive correlation (both assets move in the same direction), while -1 indicates a perfect negative correlation (assets move in opposite directions). A correlation closer to 0 suggests little to no linear relationship between the price movements of the two assets.
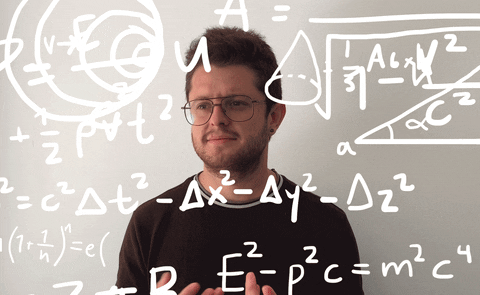
Let's say you develop a trading strategy based on a technical indicator that works well for a specific stock, like Apple (AAPL).
You might be tempted to backtest your strategy on other large-cap technology stocks like Microsoft (MSFT) or Alphabet (GOOGL). These stocks are likely to be highly correlated with Apple, meaning their prices tend to move in the same direction.
However, the problem with such correlated assets is that they give a false sense of Diversification. Even though you're including multiple assets, their price movements are highly dependent on each other.
How do we cater to this problem?
To achieve true Diversification in your Backtesting, consider including assets with a lower correlation to Apple:
For Example, Adding a US Treasury bond (e.g., TLT) to your backtesting data introduces an asset with a typically low correlation to stocks. This is because bonds generally move in the opposite direction of stocks during economic downturns.
Similarly,
Including a commodity like gold (GLD) can further diversify your Backtesting. Gold prices can sometimes move independently of stocks, especially during periods of high inflation or economic uncertainty.
Or,
What you can also do is Instead of focusing solely on technology stocks, consider including assets from a different sector, like a consumer staples stock such as Procter & Gamble (PG). These companies often see steady demand for their products regardless of the overall market climate.
And Last,
5) It Validates Out of Sample data:
Out-of-sample testing involves applying your strategy to a separate data set not used in the original backtest. This provides further confirmation of your strategy's effectiveness in unseen market conditions.
Why is Out-of-Sample Validation Crucial?
Because It Combats Overfitting, as explained in our previous blog about crucial backtesting mistakes, overfitting occurs when the strategy memorizes historical patterns that might not repeat in the future, resulting in unrealistic performance expectations. Out-of-sample testing exposes the strategy to new data, revealing its ability to adapt and generalize to unseen market conditions.
How does Out-of-sample Validation work?
Let's say your swing trading strategy based on moving averages performed well in the past five years of Apple (AAPL) stock data (your in-sample data). Now, you decide to test it out-of-sample.
So, You divide your entire Apple stock data into two sets. For example, You can use the first five years for in-sample Backtesting and the most recent three years (unseen data) for out-of-sample testing.
You can then run your moving average crossover strategy on the out-of-sample data (last three years).
Then, compare your strategy's performance on the out-of-sample data with its performance on the in-sample data. Ideally, you would see similar positive results.
What the comparative Results may tell you?
If your strategy shows positive results on both the in-sample and out-of-sample data, it provides greater confidence in its potential effectiveness in real-world trading.
But,
Suppose the strategy's performance significantly differs between the in-sample and out-of-sample data. In that case, it might be overfitted to the in-sample data or might need to be more robust for changing market conditions. This would be a signal to refine your strategy before risking real capital.
In Conclusion
Remember, Backtesting is an ongoing exploration, not a one-time test. As you gain experience and market knowledge, revisit your backtesting data and refine your strategy accordingly. Embrace the journey of continuous learning, and watch your Backtesting become the springboard that propels you toward trading success.
Trade Smarter!